The Complete Guide to Moving Averages: Types and Their Trading Applications
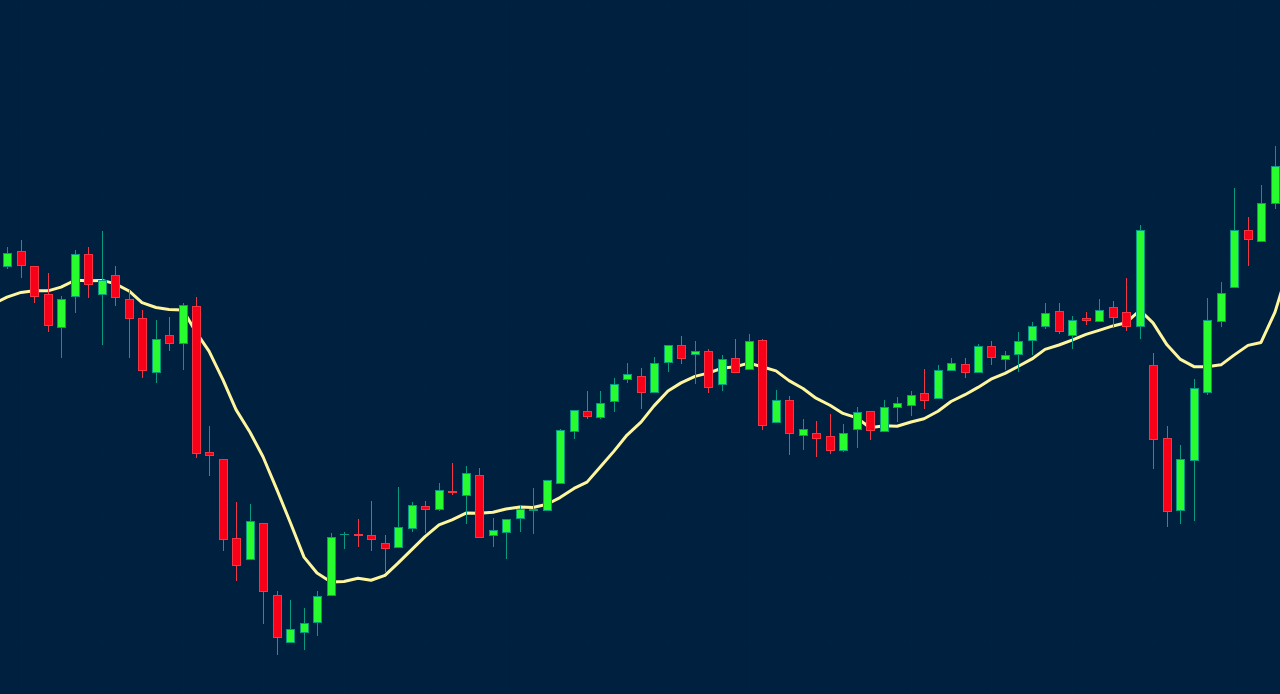
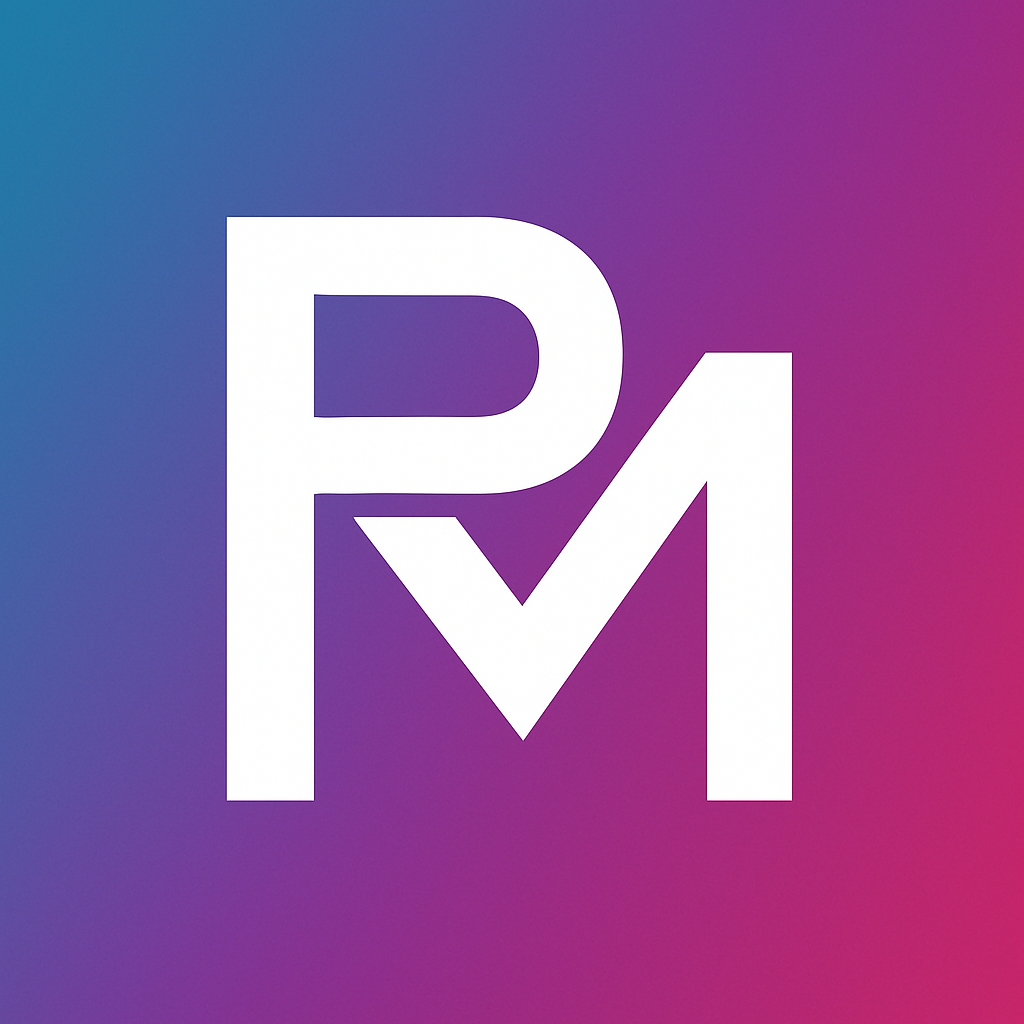
What are Moving Averages?
Moving Averages (MAs) are cornerstone technical indicators used to smooth out price action and identify trend direction over a specific timeframe. By calculating the average price of an asset over a defined number of periods (candles), MAs filter out short-term noise to provide a clearer view of the underlying market direction. As lagging indicators, they react to past price movements rather than predicting future ones, making them invaluable for trend confirmation and identifying potential support or resistance levels.
While the concept is straightforward, there's remarkable variety in how different MA types calculate their values. From the simple arithmetic mean of an SMA to the complex adaptive calculations of KAMA, each MA type offers distinct advantages for specific market conditions and trading styles. Understanding these differences enables traders to select the most appropriate MA type for their particular strategy and market environment.
Table of Contents
- Simple Moving Average (SMA)
- Exponential Moving Average (EMA)
- Weighted Moving Average (WMA)
- Smoothed Moving Average (SMMA)
- Linear Weighted Moving Average (LWMA)
- Double Exponential Moving Average (DEMA)
- Triple Exponential Moving Average (TEMA)
- Hull Moving Average (HMA)
- Adaptive Moving Average (AMA)
- Triangular Moving Average (TMA)
- Kaufman Adaptive Moving Average (KAMA)
- Variable Index Dynamic Average (VIDYA)
- Linear Regression Moving Average (LRMA)
- Displaced Moving Average (DMA)
- Time Series Forecast (TSF)
- Wilder's Moving Average
- Zero Lag Exponential Moving Average (ZLEMA)
- Fractal Adaptive Moving Average (FRAMA)
- McGinley Dynamic
- Time Weighted Average Price (TWAP)
- Arnaud Legoux Moving Average (ALMA)
- Volatility Adjusted Moving Average (VAMA)
- Trend Regularity Adaptive Moving Average (TRAMA)
- Volume Weighted Moving Average (VWMA)
- Volume Weighted Average Price (VWAP)
- Gaussian Moving Average (GMA)
- Jurik Moving Average (JMA)
- T3 Moving Average (T3)
Types of Moving Averages
Simple Moving Average (SMA)
The most basic and widely used MA type. It calculates the arithmetic mean of prices over a specific period (n), giving equal weight to each data point. SMA is valued for its simplicity and effectiveness in identifying broader trends, though it responds more slowly to price changes compared to other MA types.
Formula:
SMA(n) = (P₁ + P₂ + ... + Pₙ) / n Alternatively, in summation notation: SMA(n) = (1/n) × ∑ᵢ₌₁ⁿ Pᵢ
Where P = Price (typically close price) for a period, n = Number of periods. SMA gives equal importance to all price points within the calculation period. When a new period forms, the oldest price is dropped and the newest is added.
Exponential Moving Average (EMA)
Gives greater weight to more recent prices using an exponentially weighted multiplier (α), making it more responsive to recent price changes than the SMA. This responsiveness helps traders identify trend changes more quickly, but may lead to more false signals in volatile markets.
Formula:
EMA = Price × α + Previous_EMA × (1 - α) Where α = 2 / (n + 1) Recursively: EMA(t) = α × Price(t) + (1 - α) × EMA(t-1)
The first EMA value is typically the SMA(n). EMA places approximately 86% of its weight on data from the last 2n periods. The formula can also be written as: EMA = Previous_EMA + α × (Price - Previous_EMA), which shows how EMA adjusts based on the error between current price and previous average.
Weighted Moving Average (WMA)
Assigns a linearly increasing weight to prices, with the most recent price (Pₙ) having the highest weight (n). It reacts faster than SMA but typically slower than EMA. WMA provides a good balance between responsiveness and smoothness, making it popular for intermediate-term trend analysis.
Formula:
WMA = [(P₁ × 1) + (P₂ × 2) + ... + (Pₙ × n)] / [n(n+1)/2] In summation form: WMA = ∑ᵢ₌₁ⁿ (Pᵢ × i) / ∑ᵢ₌₁ⁿ i
The denominator [n(n+1)/2] is the sum of weights (1 + 2 + ... + n). For example, in a 5-period WMA, weights would be 1, 2, 3, 4, and 5, summing to 15. The most recent price (Pₙ) receives n times more weight than the oldest price (P₁).
Smoothed Moving Average (SMMA)
Considers all historical data, assigning exponentially decreasing weight similar to EMA but with a different smoothing logic. SMMA moves slower than EMA/SMA and provides exceptionally smooth curves, making it excellent for long-term trend identification and reducing noise in volatile markets.
Formula:
SMMA(t) = [Previous_SMMA × (n - 1) + Price(t)] / n Equivalently: SMMA(t) = SMMA(t-1) - [SMMA(t-1) / n] + [Price(t) / n]
First SMMA = SMA(n). The recursive calculation inherently considers long history, giving SMMA a longer 'memory' of price action than most other moving averages. SMMA is equivalent to an EMA with α = 1/n, similar to Wilder's MA.
Linear Weighted Moving Average (LWMA)
Functionally identical to the Weighted Moving Average (WMA). Assigns linearly increasing weight to recent prices, prioritizing recent market activity while still considering older data. Some platforms might implement subtle variations, but the core concept remains the same.
Formula:
LWMA = [(P₁ × 1) + (P₂ × 2) + ... + (Pₙ × n)] / [n(n+1)/2] In summation form: LWMA = ∑ᵢ₌₁ⁿ (Pᵢ × i) / ∑ᵢ₌₁ⁿ i
LWMA and WMA calculate the same value in most implementations. The term LWMA is sometimes used to emphasize the linear nature of the weighting. In some platform-specific implementations, subtle differences in normalization might exist.
Double Exponential Moving Average (DEMA)
Developed by Patrick Mulloy, DEMA attempts to reduce EMA lag by applying an EMA to the price and then applying another EMA to the result, combining them to cancel out lag. This results in a more responsive indicator that follows price more closely than standard EMAs, particularly useful in trending markets.
Formula:
DEMA(n) = 2 × EMA(Price, n) - EMA(EMA(Price, n), n) Alternative form: DEMA(n) = EMA(Price, n) + [EMA(Price, n) - EMA(EMA(Price, n), n)]
DEMA requires calculating an EMA and an EMA of that EMA. Despite its name, it's not simply an EMA of an EMA (which would increase lag), but rather a specific formula designed to reduce lag. The alternative form shows how DEMA is effectively an EMA plus a component that represents the lag [EMA - EMA(EMA)].
Triple Exponential Moving Average (TEMA)
Also developed by Patrick Mulloy, TEMA extends DEMA by using a triple-smoothed EMA to further reduce lag, making it even more responsive. TEMA is particularly effective in trending markets and for shorter timeframes where quick signal generation is important, though it can be erratic in sideways markets.
Formula:
TEMA(n) = 3 × EMA(Price, n) - 3 × EMA(EMA(Price, n), n) + EMA(EMA(EMA(Price, n), n), n) Alternative form: TEMA(n) = EMA(n) + [EMA(n) - EMA(EMA(n))] + [EMA(n) - 2×EMA(EMA(n)) + EMA(EMA(EMA(n)))]
TEMA requires calculating EMA, EMA of EMA, and EMA of EMA of EMA. Like DEMA, it's not simply applying EMA three times but uses a specific formula designed to minimize lag. The pattern follows from DEMA, with each term helping cancel out more lag from the previous terms.
Hull Moving Average (HMA)
Designed by Alan Hull to provide significant lag reduction while maintaining smoothness. The HMA virtually eliminates lag by using weighted moving averages and dampening the smoothing effect. It produces a remarkably responsive yet smooth curve that tracks price activity closely.
Formula:
HMA(n) = WMA( [2 × WMA(Price, n/2)] - WMA(Price, n), sqrt(n) ) Step by step: 1. Calculate WMA(Price, n/2) 2. Calculate WMA(Price, n) 3. Calculate 2 × WMA(Price, n/2) - WMA(Price, n) 4. Calculate WMA of the result from step 3 with period sqrt(n)
Integer values are typically used for n/2 and sqrt(n). For example, with n=16, you would calculate WMA(8), WMA(16), and then a final WMA(4) where 4 is sqrt(16). The HMA involves calculating two WMAs and then a third WMA on their difference. The 2× multiplier on the first WMA term overemphasizes the shorter-period WMA to reduce lag.
Adaptive Moving Average (AMA)
A general term for MAs that adjust their smoothing factor based on market volatility or trend efficiency. AMAs become more responsive during trending markets and more stable during ranging markets, automatically adapting to changing market conditions without requiring parameter changes from the trader.
Formula:
AMA(t) = AMA(t-1) + α(t) × [Price(t) - AMA(t-1)] Where α(t) is a variable smoothing factor determined by market conditions, typically between a fast_α and slow_α value.
Specific implementations like KAMA and VIDYA are types of AMAs. Each uses different methods to calculate the adaptive factor based on price volatility or directional movement consistency. The core concept is that the smoothing factor changes dynamically rather than remaining fixed as in EMA.
Triangular Moving Average (TMA)
A double-smoothed SMA. Effectively applies an SMA to the result of another SMA, giving highest weight to the middle of the period. TMAs produce an extremely smooth line that eliminates much of the market noise, making them excellent for identifying the primary trend in choppy markets, though with significant lag.
Formula:
TMA(n) = SMA( SMA(Price, ceil((n+1)/2)), floor((n+1)/2) ) For even n: TMA(n) = SMA( SMA(Price, n/2+1), n/2 ) For odd n: TMA(n) = SMA( SMA(Price, (n+1)/2), (n+1)/2 )
Very smooth but lags considerably. For example, a 10-period TMA would first calculate a 6-period SMA (10/2+1), then calculate a 5-period SMA (10/2) of that result. The weight distribution forms a triangular pattern, with middle values receiving greatest weight. A 10-period TMA has a triangular weighting pattern: 1,2,3,4,5,5,4,3,2,1.
Kaufman Adaptive Moving Average (KAMA)
A responsive moving average that adjusts its smoothing based on market volatility. In trending markets, KAMA follows price closely, while in choppy markets, it filters out noise by moving more slowly.
Formula:
KAMA(n)(t) = KAMA(n)(t-1) + SC × (Price(t) - KAMA(n)(t-1)) Where: SC = [ER × (0.666 - 0.0645) + 0.0645]² ER = Efficiency Ratio = |Price(t) - Price(t-n)| / Sum(|Price(i) - Price(i-1)|) for i from t-n+1 to t
KAMA's efficiency ratio determines how responsive the average will be. Higher efficiency (strong trend) results in faster following of price, while lower efficiency (choppy market) leads to more smoothing. KAMA excels at adapting to different market conditions without requiring parameter changes.
Variable Index Dynamic Average (VIDYA)
Developed by Tushar Chande, VIDYA uses a volatility index (like CMO or Standard Deviation) to dynamically adjust the EMA smoothing factor. It responds quickly in trending markets and slows down in ranging markets, providing adaptive signals based on the current market condition.
Formula:
VIDYA(t) = Price(t) × α × VI + VIDYA(t-1) × (1 - α × VI) Where: α = 2/(n+1) VI = |CMO| / 100 (or another normalized volatility index between 0 and 1) CMO = [Sum(Up, n) - Sum(Down, n)] / [Sum(Up, n) + Sum(Down, n)]
VI is typically the absolute value of Chande Momentum Oscillator (CMO) divided by 100, or another normalized volatility measure between 0 and 1. Higher volatility in the direction of the trend increases responsiveness. When VI=1 (maximum), VIDYA behaves like a standard EMA with period n. When VI approaches 0, VIDYA barely changes, preserving the previous value.
Linear Regression Moving Average (LRMA)
Calculates a linear regression line over the past 'n' periods and plots the current endpoint value of that line. LRMA is based on statistical best-fit lines rather than averaging, making it theoretically optimal for minimizing the sum of squared deviations from price. It's particularly effective at capturing trends.
Formula:
LRMA(n) = a + b × n Where a and b are determined by solving: a = (∑y) / n - b × (∑x) / n b = [n(∑xy) - (∑x)(∑y)] / [n(∑x²) - (∑x)²] With x = time indices and y = prices
Also known as Linear Regression Value or End Point Moving Average (EPMA). The linear regression line minimizes the sum of squared distances between itself and actual prices, providing a statistically optimal fit to recent price action. Unlike true averaging methods, LRMA can sometimes move opposite to price in anticipation of continuing a linear trend.
Displaced Moving Average (DMA)
A standard MA (SMA, EMA, etc.) shifted forward (positive offset) or backward (negative offset) in time. Forward displacement is used for projecting potential future MA values, while backward displacement can reduce lag by effectively 'predicting' where the MA would be based on historical behavior.
Formula:
DMA(t, n, offset) = MA(Price, n)(t+offset) For example: DMA with offset = -5 plots MA(t-5) at time t DMA with offset = +5 plots MA(t+5) at time t
For example, MA(10)[-5] would plot the 10-period MA shifted 5 periods earlier, reducing lag. MA(10)[+5] would project the MA 5 periods into the future, useful for anticipated support/resistance. Forward-displaced MAs (positive offset) for future periods use known price data to 'predict' where the MA would be if prices stayed constant.
Time Series Forecast (TSF)
Calculates a linear regression line over 'n' periods and plots the value projected one period into the future based on that line's slope. TSF is essentially a forward-displaced LRMA that attempts to forecast where price would be if it continued along the regression line.
Formula:
TSF(n)(t) = LRMA(n)(t+1) = a + b × (n+1) Where a and b are the intercept and slope of the linear regression line over past n periods, as computed for LRMA.
TSF projects the linear regression line one period forward. It's particularly useful for anticipating potential price targets if the current trend continues. TSF can be thought of as a DMA with a displacement of +1 applied to an LRMA. Sensitivity to trends increases with smaller values of n, while larger values provide smoother forecasts.
Wilder's Moving Average
A specific type of smoothed MA developed by J. Welles Wilder Jr. and used in his indicators like RSI and ADX. Similar to EMA but uses a smoothing factor of 1/n instead of 2/(n+1), resulting in a smoother line that responds more slowly to price changes.
Formula:
WilderMA(t) = WilderMA(t-1) + [Price(t) - WilderMA(t-1)] / n Alternatively: WilderMA(t) = [Price(t) + (n-1) × WilderMA(t-1)] / n
Equivalent to an EMA with α = 1/n, which is approximately equivalent to an EMA(2n-1). Sometimes called RMA (Running Moving Average) in some platforms and languages like Pine Script. For example, Wilder's 14-day MA is roughly equivalent to a 27-day EMA (2×14-1). The first value is typically an SMA(n) of initial data.
Zero Lag Exponential Moving Average (ZLEMA)
Developed by John Ehlers, ZLEMA aims to eliminate lag by incorporating a de-lagging element based on the difference between the current price and a delayed price. This approach effectively removes the cumulative lag effect present in traditional EMAs.
Formula:
ZLEMA(t) = EMA(2 × Price(t) - Price(t-lag), n) Where lag = (n-1)/2 (rounded to nearest integer) Alternatively: data = Price(t) + [Price(t) - Price(t-lag)] ZLEMA(t) = EMA(data, n)
The lag parameter is typically floor((n-1)/2). ZLEMA uses the price difference between current and lagged prices to extrapolate forward, thereby creating an effectively lag-free input to the EMA calculation. It is particularly effective in trending markets while maintaining smoothness. ZLEMA generally reacts faster than DEMA and TEMA with the same period setting.
Fractal Adaptive Moving Average (FRAMA)
Developed by John Ehlers, FRAMA adapts its smoothing based on the fractal dimension of price movement. It uses fractal geometry principles to determine whether the market is trending or in a sideways pattern, adjusting its smoothing factor accordingly.
Formula:
FRAMA(t) = FRAMA(t-1) + α(t) × [Price(t) - FRAMA(t-1)] Where α(t) is derived from the fractal dimension (D): D = (log(N1+N2) - log(N3)) / log(2) α(t) = exp(-4.6 × (D-1)) And constrained between Fast_α and Slow_α
N1, N2, and N3 are price ranges of different segments used to calculate the fractal dimension D. The dimension approaches 1 in a trending market and 2 in a sideways market. α(t) is typically constrained between 0.01 (slow) and 0.5 (fast). FRAMA reacts very quickly to strong trends while providing significant smoothing in choppy markets.
McGinley Dynamic
Developed by John McGinley as an improvement over traditional moving averages. It incorporates a dynamic smoothing factor that adjusts based on the speed of price movement, resulting in faster tracking during trends and less whipsaws in consolidation.
Formula:
MD(t) = MD(t-1) + [Price(t) - MD(t-1)] / [N × (Price(t)/MD(t-1))⁴] Where N is the period parameter
The McGinley Dynamic stays closer to price in strong trends and further from price in consolidations. The fourth power in the denominator ensures significant smoothing for small price changes but accelerates tracking for larger moves. Unlike conventional MAs, McGinley Dynamic doesn't need optimization for different markets or timeframes. Typical values for N range from 10 to 40.
Time Weighted Average Price (TWAP)
TWAP places greater weight on more recent prices based on the exact time elapsed, unlike regular moving averages that weight by periods. It's particularly useful in algorithmic trading and order execution where the time dimension is critical for capturing intraday price dynamics.
Formula:
TWAP = ∑(Price × Time_Weight) / ∑Time_Weight Where Time_Weight is proportional to the recency of the data point, often calculated as: Time_Weight(i) = (Current_Time - Start_Time) - (Time(i) - Start_Time)
TWAP is often used by institutional traders for order execution to minimize market impact. It differs from traditional period-based MAs by considering the actual timestamps of data points rather than just their sequence. TWAP orders are executed evenly over a specified time window to achieve an average execution price close to the time-weighted average price of the asset during that window.
Arnaud Legoux Moving Average (ALMA)
Developed by Arnaud Legoux and Dimitrios Kouzis-Loukas, ALMA combines a Gaussian distribution with an offset parameter to create a moving average with minimal lag and noise reduction. It's designed to filter market noise while maintaining responsiveness to genuine price movements.
Formula:
ALMA(n) = ∑(Price × Weight) / ∑Weight Where: Weight(i) = exp(-((i - offset × (n-1))²) / (2 × sigma² × (n-1)²)) offset ∈ [0, 1] controls the applied offset (0.85 default) sigma controls the filter width (6 default)
The offset parameter (typically 0.85) shifts the Gaussian weight distribution to reduce lag. When offset=1, the weights are shifted to emphasize the most recent prices, reducing lag but potentially increasing noise. When offset=0, the weights are centered, maximizing smoothness but increasing lag. The sigma parameter (typically 6) controls the width of the Gaussian curve, with smaller values creating a narrower distribution focused on fewer prices.
Volatility Adjusted Moving Average (VAMA)
VAMA adapts to market volatility by adjusting the length of the moving average window based on current market conditions. During high volatility, it shortens the calculation period to respond more quickly; during low volatility, it lengthens the period for greater smoothing.
Formula:
VAMA(t) = SMA(Price, Adjusted_Length(t)) Where: Adjusted_Length(t) = Base_Length × Volatility_Ratio(t) Volatility_Ratio(t) = Long_Term_Volatility / Short_Term_Volatility Volatility can be measured using Average True Range (ATR) or Standard Deviation
VAMA automatically adjusts to market conditions without requiring parameter changes from the trader. During high volatility periods (when short-term volatility exceeds long-term volatility), the effective length decreases, making VAMA more responsive. During low volatility or ranging markets, the effective length increases, producing smoother output with fewer false signals. The specific implementation can vary, with some versions using ATR ratios and others using standard deviation comparisons.
Trend Regularity Adaptive Moving Average (TRAMA)
TRAMA adapts to the regularity of market trends by analyzing the fractal dimension and directional strength of price movements. It dynamically adjusts its responsiveness based on how 'trending' the market is, providing faster signals in strong trends and more filtering in choppy conditions.
Formula:
TRAMA(t) = TRAMA(t-1) + α(t) × [Price(t) - TRAMA(t-1)] Where α(t) is determined by the Trend Regularity Index (TRI): TRI = 1 - Fractal_Dimension / 2 α(t) = Min_Alpha + (Max_Alpha - Min_Alpha) × TRI Fractal_Dimension is calculated similarly to FRAMA
TRAMA builds on concepts similar to FRAMA but incorporates additional directional strength measures. The Trend Regularity Index (TRI) approaches 0.5 in strong trends and 0 in completely random markets. This adjusts the smoothing factor α between its minimum and maximum values accordingly. TRAMA is particularly effective at filtering out noise during consolidation while maintaining responsiveness during trending markets. It often outperforms other adaptive MAs in markets that alternate between trending and ranging behavior.
Volume Weighted Moving Average (VWMA)
Incorporates volume data along with price, giving more weight to price points with higher trading volume. This makes VWMA particularly useful for identifying significant support/resistance levels where high volume confirms price action.
Formula:
VWMA(n) = ∑(Price × Volume) / ∑Volume In expanded form: VWMA(n) = [(P₁×V₁) + (P₂×V₂) + ... + (Pₙ×Vₙ)] / (V₁ + V₂ + ... + Vₙ)
VWMA gives greater importance to prices with higher volume, effectively reflecting the average price at which the asset was traded over the period, rather than just the arithmetic average of prices. It's particularly useful in markets where volume precedes price movement, and for identifying stronger support/resistance levels where significant volume has occurred.
Volume Weighted Average Price (VWAP)
A special case of VWMA that calculates from the beginning of the trading session (typically daily). VWAP represents the true average price at which an asset has traded throughout the current session, weighted by volume.
Formula:
VWAP = ∑(Price × Volume) / ∑Volume Where the summation runs from the start of the current session to the present time
Unlike other MAs, VWAP typically resets at the beginning of each trading session. It's widely used by institutional traders to determine optimal entry and exit points. Prices above VWAP generally indicate bullish sentiment for the session, while prices below suggest bearish sentiment. Multiple-day VWAPs are also used, with 3-day, 5-day, and 20-day being common.
Gaussian Moving Average (GMA)
Applies a Gaussian (normal) distribution of weights to price data, with the center of the distribution receiving the highest weight. GMA produces an exceptionally smooth curve with minimal lag compared to other highly smoothed averages.
Formula:
GMA = ∑(Price × Gaussian_Weight) / ∑Gaussian_Weight Where Gaussian_Weight = e^(-(i-center)²/(2×σ²)) σ is the standard deviation parameter center = (n-1)/2
The parameter σ controls the 'spread' of the Gaussian bell curve. Lower values create narrower distributions focusing weight on the center points. GMA produces smoother lines than SMA with similar lag characteristics, making it useful for trend identification in noisy data. The Gaussian weighting results in a more natural transition than linear or exponential weights.
Jurik Moving Average (JMA)
A proprietary moving average developed by Mark Jurik, designed to provide significant noise reduction while maintaining responsiveness to genuine price movements. JMA uses sophisticated adaptive techniques to optimize its performance across different market conditions.
Formula:
The exact formula is proprietary, but JMA uses multiple phases: 1. Price series smoothing with variable-length processing 2. Adaptive mechanisms to detect trends vs. noise 3. Dynamic phase-shift compensation to reduce lag 4. Smoothness control based on market volatility
JMA is known for its smooth output and reduced lag compared to traditional MAs. It has three main parameters: length (period), phase (-100 to 100, controlling lag vs. smoothness), and power (1 to 6, controlling adaptiveness). Despite its complexity, JMA is valued for consistent performance across different timeframes and market conditions.
T3 Moving Average (T3)
Developed by Tim Tillson, the T3 is a sophisticated triple EMA with an additional volume factor parameter. It provides significant smoothing while maintaining responsiveness and reducing lag through multiple EMA applications with optimized coefficients.
Formula:
T3 = c1×EMA1 + c2×EMA2 + c3×EMA3 + c4×EMA4 + c5×EMA5 + c6×EMA6 Where: EMA1 = EMA(Price) EMA2 = EMA(EMA1) EMA3 = EMA(EMA2) EMA4 = EMA(EMA3) EMA5 = EMA(EMA4) EMA6 = EMA(EMA5) And coefficients ci are derived from volume factor 'a'
The volume factor 'a' typically ranges from 0 to 1, with 0.7 being the recommended value by Tillson. The coefficients are calculated as: c1 = -a³, c2 = 3a² + 3a³, c3 = -6a² - 3a - 3a³, c4 = 1 + 3a + a³ + 3a², c5 = 3a + 3a², c6 = a³. T3 with a=0 becomes a simple EMA, while a=1 creates maximum smoothing.
How to Use Moving Averages in Trading
Moving averages are versatile tools used in various trading strategies:
- Trend Identification: The slope of an MA indicates the trend direction. An upward slope suggests an uptrend, while a downward slope suggests a downtrend. Price consistently above the MA confirms bullishness; below confirms bearishness.
- Support and Resistance: In trends, MAs often act as dynamic support (in uptrends) or resistance (in downtrends) levels where price might react.
- Crossovers: Using two MAs of different lengths (e.g., 50-period and 200-period), traders watch for crossovers. A shorter MA crossing above a longer MA (Golden Cross) is often seen as bullish, while a cross below (Death Cross) is considered bearish.
- Signal Generation: Price crossing above or below an MA can be used as a basic entry or exit signal, especially when aligned with the broader trend.
- Volatility Assessment: The distance between price and its MA can indicate market volatility or potential overbought/oversold conditions.
- Multiple Timeframe Analysis: Applying the same MA type across different timeframes helps identify confluences of support/resistance and trend alignment.
Choosing the Right MA and Period
The best MA type and period depend on your trading style, timeframe, and the market conditions:
- SMA: Excellent for identifying long-term trends and major support/resistance zones due to its smooth nature. Popular periods include 50, 100, and 200.
- EMA/WMA/LWMA: Better for short-term trading or strategies requiring faster signals, as they react quicker to recent price action. Common periods range from 5 to 20.
- DEMA/TEMA/HMA: Ideal for very short-term trading or scalping where minimizing lag is crucial. These can generate earlier signals but may create more noise in choppy markets.
- Adaptive MAs (KAMA, VIDYA): Most useful in markets that alternate between trending and ranging conditions, as they automatically adjust responsiveness without requiring parameter changes.
- Periods: Shorter periods (5-20) track price closely and generate frequent signals; medium periods (21-50) identify intermediate trends; longer periods (100-200) define major market trends and significant support/resistance zones.
Conclusion
Moving Averages represent one of the most versatile and widely-used families of technical indicators. From the straightforward SMA to the complex adaptive algorithms of KAMA or VIDYA, each variant offers unique characteristics suited to specific market conditions and trading objectives. The key to effective MA implementation lies in understanding not just how each type calculates its values, but how those calculations translate to practical trading applications.
For beginners, starting with simple MAs like SMA or EMA provides a solid foundation for understanding trend direction and potential support/resistance levels. As traders advance, exploring lag-reduced options like DEMA, TEMA, and HMA can enhance signal timing, while adaptive variants offer sophisticated responsiveness to changing market conditions without requiring constant parameter adjustments.
Ultimately, no single MA type is universally superior—each excels in specific contexts. The most effective approach involves selecting MA types and parameters aligned with your trading timeframe, strategy objectives, and the characteristics of the markets you trade. Many successful traders combine multiple MA types to leverage their complementary strengths, creating robust systems that perform well across diverse market conditions.